Many women with ovarian cancer initially respond to chemotherapy but eventually relapse because their cancer becomes resistant to standard treatment. This occurs for a variety of reasons, including:
- slow and insufficient immune response
- T lymphocytes (T cells) – the body’s cancer- and infection-fighting cells – becoming “exhausted”
- tumors which recruit cells and other factors that actually prevent the immune system from working properly
- a suppressive tumor environment which makes it difficult for drugs and immune cells to access the cancer cells they are targeting
- a failure by dendritic cells (DC) to present the correct information to T cells to enable them to find and destroy cancer cells.
The two individual research projects (IRP) being conducted as part of the Roswell Park-University of Chicago Ovarian Cancer SPORE aim to make the immune system work better against ovarian cancer.
IRP 1: Reprogramming the immunosuppressive ovarian TME with OV and a CXCR4 antagonist
Co-Leaders: Danuta Kozbor, PhD (Basic); Kunle Odunsi, MD, PhD (Clinical)
Brief summary
The goal of IRP1 is to test in a phase I/II clinical trial an innovative viral therapy administered directly into the site of the tumor, in combination with standard chemotherapy and immunotherapy. The hope is that the viral therapy will reverse the immunosuppressive tumor microenvironment and stimulate better T cell responses, thereby providing a new treatment option for women with chemotherapy-resistant ovarian cancer. Importantly, administering the treatment directly to the site of the tumor is expected to reduce the risk of systemic toxicities.
Detailed summary
The goal of our studies is to generate efficacious tumor-specific T cell responses in patients with chemotherapy-resistant high-grade serous ovarian cancer (HGSOC). Key stumbling blocks underpinning immunotherapy resistance against HGSOC include:
- insufficient expansion of tumor antigen-specific T cells
- recruitment of T regulatory and myeloid-derived suppressor cells via tumor CXCL12 production
- exhaustion of tumor-infiltrating T lymphocytes (TILs) associated with upregulation of PD1
- low tumor immunogenicity due to reduced mutation load and IFNβ production
- insufficient recruitment of intratumoral dendritic cells
- disorganized tumor vasculature incapable of supporting TIL trafficking
Herein, we propose to target these immune resistance mechanisms using an innovative, clinically translatable viral oncotherapy combination for effective direct oncolysis and reprogramming antitumor immunity.
The scientific premise of this project stems from our discovery that blockade of the CXCL12 chemokine/CXCR4 receptor axis in the chemotherapy-resistant ovarian cancer by intraperitoneal delivery of an oncolytic vaccinia virus expressing a CXCR4 antagonist (OVV-CXCR4-A-Fc) in combination with pegylated liposomal doxorubicin (PLD) yields a significant therapeutic impact by reversing the immunosuppressive TME and stimulating rigorous T cell responses (depicted in the graphic abstract below). Our proposed, mechanistic studies conducted in a phase I/II clinical trial will test the hypothesis that in patients receiving liposomal doxorubicin for platinum-resistant/refractory HGSOC, in vivo tumor destruction by OVV-CXCR4-A-Fc will provide clinical benefit by:
- abrogating tumor immune suppression
- promoting the accumulation of TILs
- when combined with PDL1 blockade, limiting T cell exhaustion
The approach is to first determine whether intraperitoneal OVV-CXCR4-A-Fc safely transforms the ovarian TME from tolerogenic to immunogenic in a first-in-human clinical trial in patients with platinum-resistant/refractory HGSOC. Second, we will establish whether OVV-CXCR4-A-Fc combined with anti-PDL1 antibody treatment is safe and clinically efficacious. Third, we will test whether the combinatorial regimen generates functionally distinct tumor-specific CD8+ and CD4+ effector/memory cells. Finally, we plan to uncover the molecular mechanisms by which OVV-CXCR4-A-Fc overcomes the ovarian tumor “vascular checkpoint” to enhance T cell migration and trafficking.
IRP 2: Synchronizing immunopeptidome of EOC-pulsed DC with tumor cells to ensure expansion of tumor-reactive T cells
Co-Leaders: Pawel Kalinski, MD, PhD (Applied), Song Liu, PhD (Basic), Emese Zsiros, MD, PhD, FACOG (Clinical)
Brief summary
There is a type of immune cell called a dendritic cell (DC) which gathers proteins found on the surface of cancer cells and “presents” them to T cells to help the T cells find and kill other cancer cells which have the same proteins on their surface. Sometimes, however, DCs present the wrong proteins, preventing the T cells from locating the tumor cells. The goal of IRP2 is to test a method for bypassing this information “mismatch” by loading DCs with proteins specific to the patient’s tumor, making the DCs better at teaching T cells to find and kill the patient’s tumor cells. The phase I/II clinical trial in IRP2 will commence in 2023, following preclinical studies.
Detailed summary
Project 2 tests a new immunization strategy to enhance the induction of cytotoxic lymphocytes (CTLs) against multiple patient-specific epitopes, by targeting ovarian cancer (OvCa) cells and dendritic cells (DCs). We will compare the immunopeptidomes on OvCa cells and two populations of DC specialized in CTL induction: ex vivo generated alpha-type-1-polarized (αDC1s) and endogenous conventional DCs (cDC1s) which share the inflammatory BATF3/IRF8 phenotype and elevated ability to cross-present multiple cancer-cell-associated antigens to CD8+ T cells.
Combining our unique in vitro sensitization and bioinformatics approaches, we will test the overall hypothesis that mismatch between immunopeptidomes of OvCa cells and DCs presenting antigens from cancer cells limits therapeutic effectiveness of spontaneous and vaccination-induced CTL responses. We further hypothesize that αDC1s loaded with synthetic patient-specific neoantigen peptides will bypass such mismatch, inducing CTLs particularly effective in killing OvCa tumors. We propose the following three Aims:
Specific Aim 1: Compare the antigenic specificity of human CD8+ T cells induced by DCs loaded with autologous OvCa cells, tumor-eluted peptides and patient-specific neoantigen peptides identified in silico. We hypothesize that CTLs induced by autologous cancer cell-loaded αDC1s or cDC1s contain many CTLs which are irrelevant for tumor recognition, which can be corrected by loading DCs with synthetic neoantigen peptides specific to each patient’s OvCa cells.
Specific Aim 2: Evaluate immunopeptidome differences between OvCa cells and tumor-loaded αDC1s and endogenous cDC1s and test the feasibility of their adjustment. We hypothesize that immune adjuvants and inflammatory mediators can be used to modulate APM patterns and immunopeptidomes of DCs and OvCa cells, to enhance the antigenic match between arising CTLs and autologous OvCa cells.
Specific Aim 3: Determine the feasibility, safety and clinical efficacy of αDC1 vaccines loaded with patient-specific neoantigen peptides combined with PD-1 blockade. Guided by the results of Aim 2, the patients may also receive systemic immune modulation to increase the visibility of their own OvCa cells to αDC1-induced CTLs and reduce immune suppression.
Contact us
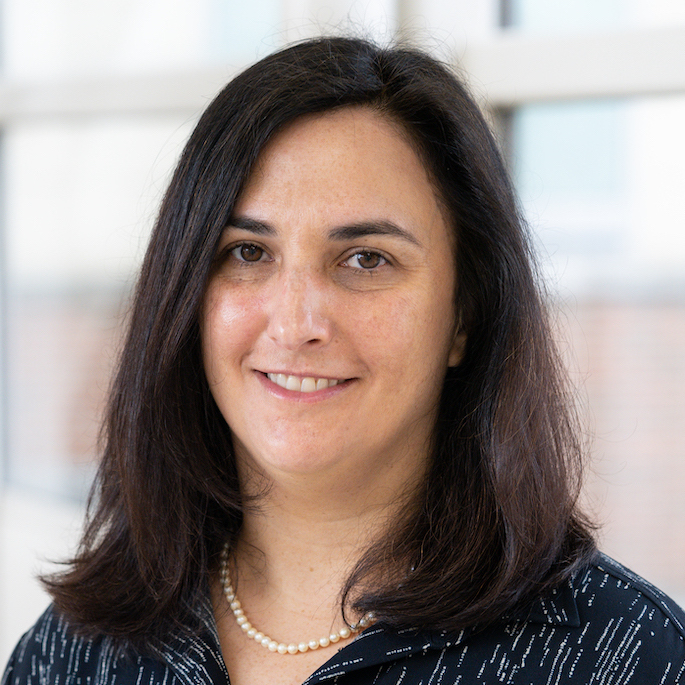